How to verify the authenticity and origin of photos and videos – Technologist
Over the past 18 months or so, we seem to have lost the ability to trust our eyes. Photoshop fakes are nothing new, of course, but the advent of generative artificial intelligence (AI) has taken fakery to a whole new level. Perhaps the first viral AI fake was the 2023 image of the Pope in a white designer puffer jacket, but since then the number of high-quality eye deceivers has skyrocketed into the many thousands. And as AI develops further, we can expect more and more convincing fake videos in the very near future.
One of the first deepfakes to go viral worldwide: the Pope sporting a trendy white puffer jacket
This will only exacerbate the already knotty problem of fake news and accompanying images. These might show a photo from one event and claim it’s from another, put people who’ve never met in the same picture, and so on.
Image and video spoofing has a direct bearing on cybersecurity. Scammers have been using fake images and videos to trick victims into parting with their cash for years. They might send you a picture of a sad puppy they claim needs help, an image of a celebrity promoting some shady schemes, or even a picture of a credit card they say belongs to someone you know. Fraudsters also use AI-generated images for profiles for catfishing on dating sites and social media.
The most sophisticated scams make use of deepfake video and audio of the victim’s boss or a relative to get them to do the scammers’ bidding. Just recently, an employee of a financial institution was duped into transferring $25 million to cybercrooks! They had set up a video call with the “CFO” and “colleagues” of the victim — all deepfakes.
So what can be done to deal with deepfakes or just plain fakes? How can they be detected? This is an extremely complex problem, but one that can be mitigated step by step — by tracing the provenance of the image.
Wait… haven’t I seen that before?
As mentioned above, there are different kinds of “fakeness”. Sometimes the image itself isn’t fake, but it’s used in a misleading way. Maybe a real photo from a warzone is passed off as being from another conflict, or a scene from a movie is presented as documentary footage. In these cases, looking for anomalies in the image itself won’t help much, but you can try searching for copies of the picture online. Luckily, we’ve got tools like Google Reverse Image Search and TinEye, which can help us do just that.
If you’ve any doubts about an image, just upload it to one of these tools and see what comes up. You might find that the same picture of a family made homeless by fire, or a group of shelter dogs, or victims of some other tragedy has been making the rounds online for years. Incidentally, when it comes to false fundraising, there are a few other red flags to watch out for besides the images themselves.
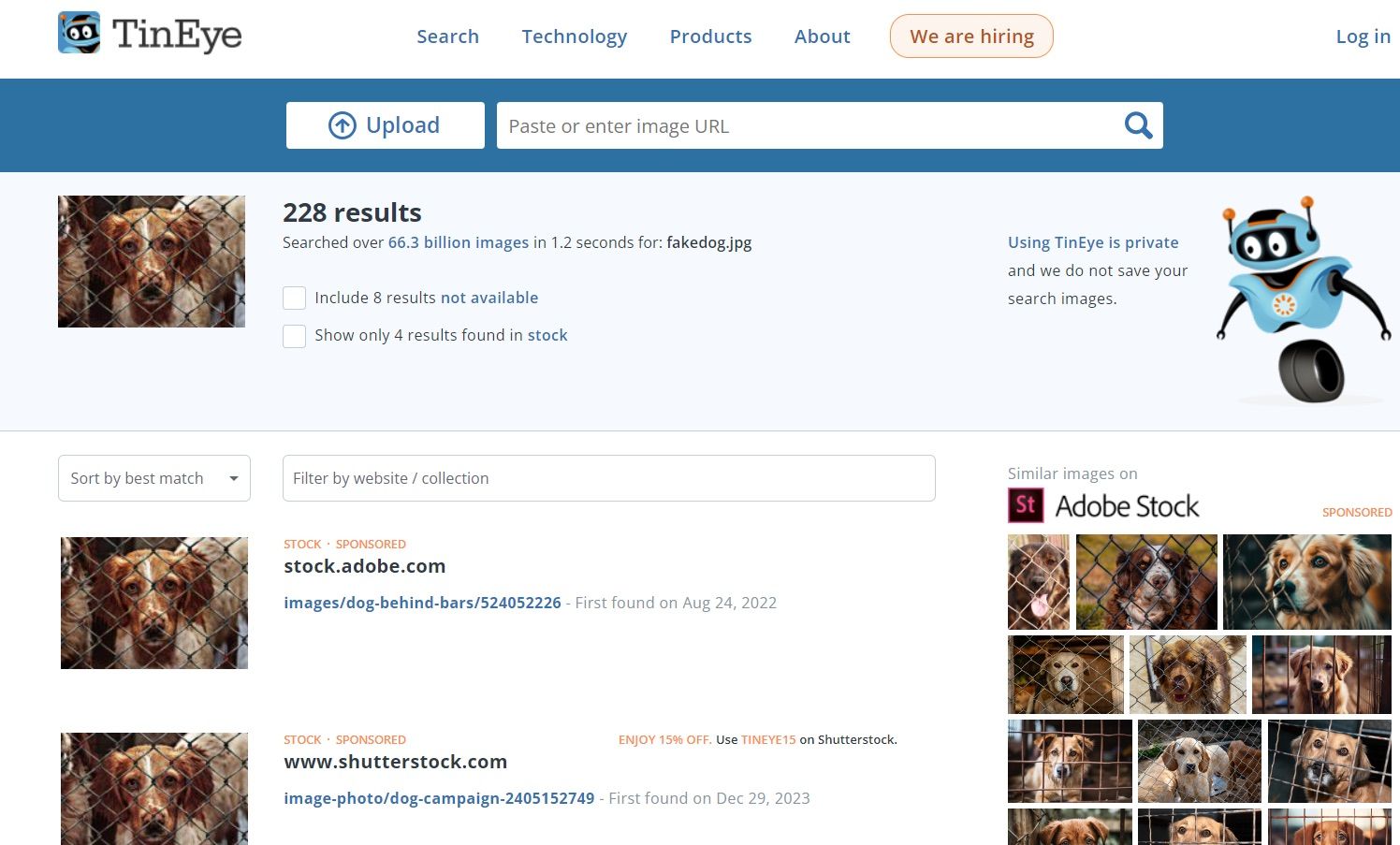
Dog from a shelter? No, from a photo stock
Photoshopped? We’ll soon know.
Since photoshopping has been around for a while, mathematicians, engineers, and image experts have long been working on ways to detect altered images automatically. Some popular methods include image metadata analysis and error level analysis (ELA), which checks for JPEG compression artifacts to identify modified portions of an image. Many popular image analysis tools, such as Fake Image Detector, apply these techniques.
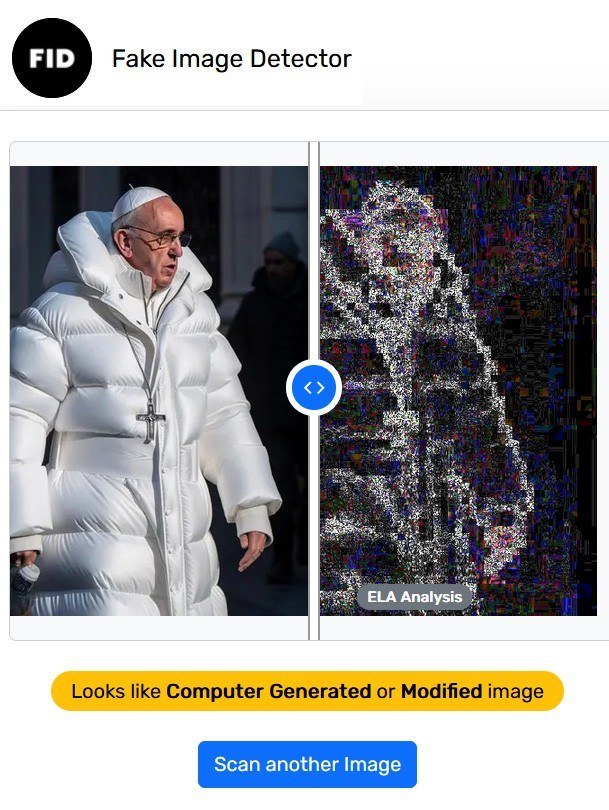
Fake Image Detector warns that the Pope probably didn’t wear this on Easter Sunday… Or ever
With the emergence of generative AI, we’ve also seen new AI-based methods for detecting generated content, but none of them are perfect. Here are some of the relevant developments: detection of face morphing; detection of AI-generated images and determining the AI model used to generate them; and an open AI model for the same purposes.
With all these approaches, the key problem is that none gives you 100% certainty about the provenance of the image, guarantees that the image is free of modifications, or makes it possible to verify any such modifications.
WWW to the rescue: verifying content provenance
Wouldn’t it be great if there were an easier way for regular users to check if an image is the real deal? Imagine clicking on a picture and seeing something like: “John took this photo with an iPhone on March 20”, “Ann cropped the edges and increased the brightness on March 22”, “Peter re-saved this image with high compression on March 23”, or “No changes were made” — and all such data would be impossible to fake. Sounds like a dream, right? Well, that’s exactly what the Coalition for Content Provenance and Authenticity (C2PA) is aiming for. C2PA includes some major players from the computer, photography, and media industries: Canon, Nikon, Sony, Adobe, AWS, Microsoft, Google, Intel, BBC, Associated Press, and about a hundred other members — basically all the companies that could have been individually involved in pretty much any step of an image’s life from creation to publication online.
The C2PA standard developed by this coalition is already out there and has even reached version 1.3, and now we’re starting to see the pieces of the industrial puzzle necessary to use it fall into place. Nikon is planning to make C2PA-compatible cameras, and the BBC has already published its first articles with verified images.
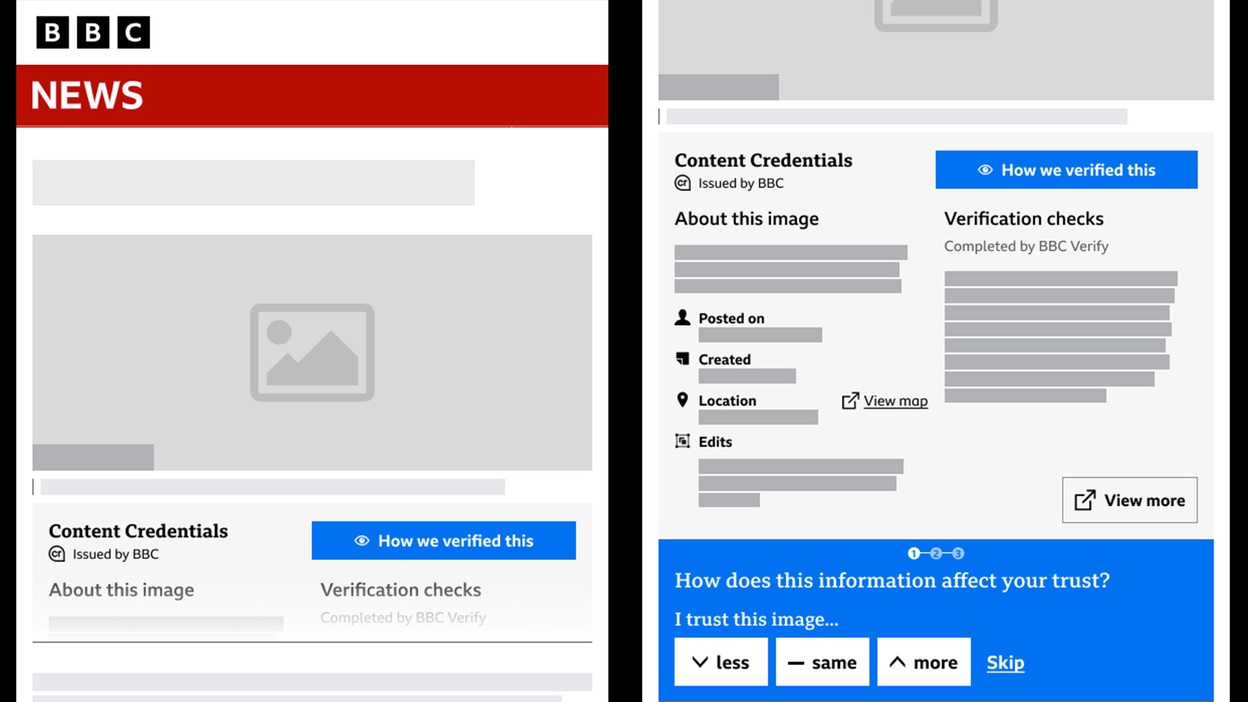
BBC talks about how images and videos in its articles are verified
The idea is that when responsible media outlets and big companies switch to publishing images in verified form, you’ll be able to check the provenance of any image directly in the browser. You’ll see a little “verified image” label, and when you click on it, a bigger window will pop up showing you what images served as the source, and what edits were made at each stage before the image appeared in the browser and by whom and when. You’ll even be able to see all the intermediate versions of the image.
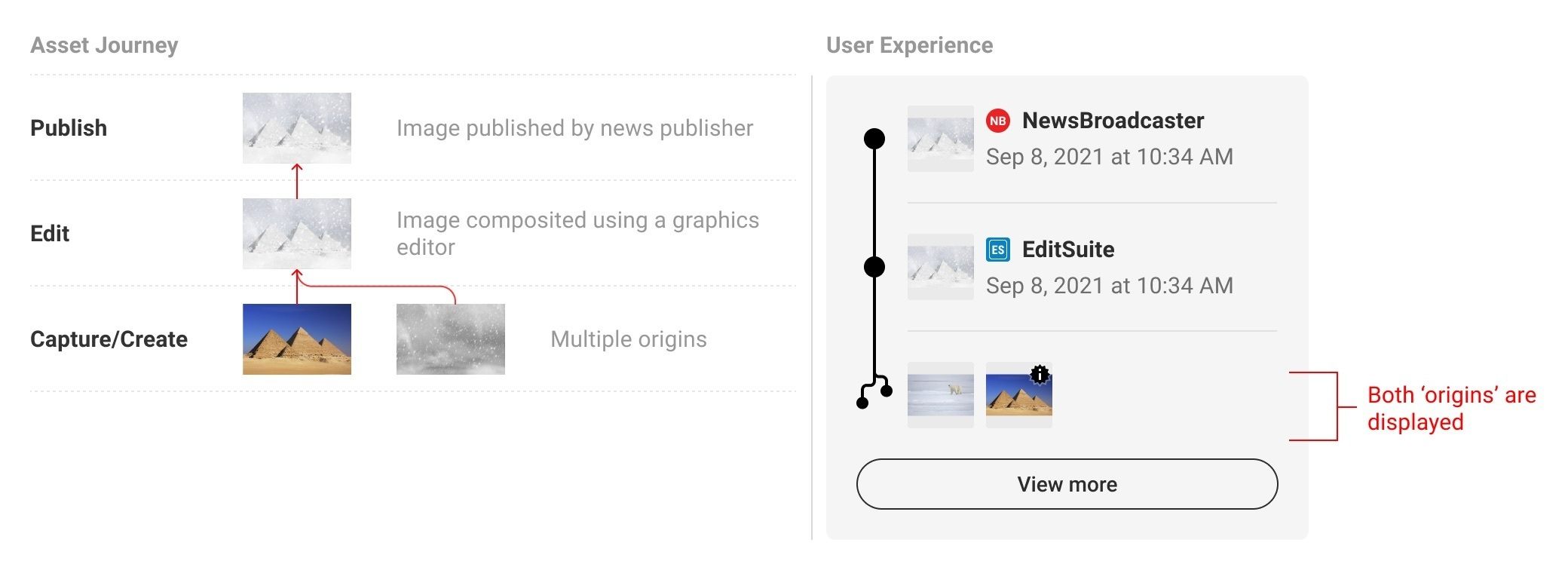
History of image creation and editing
This approach isn’t just for cameras; it can work for other ways of creating images too. Services like Dall-E and Midjourney can also label their creations.
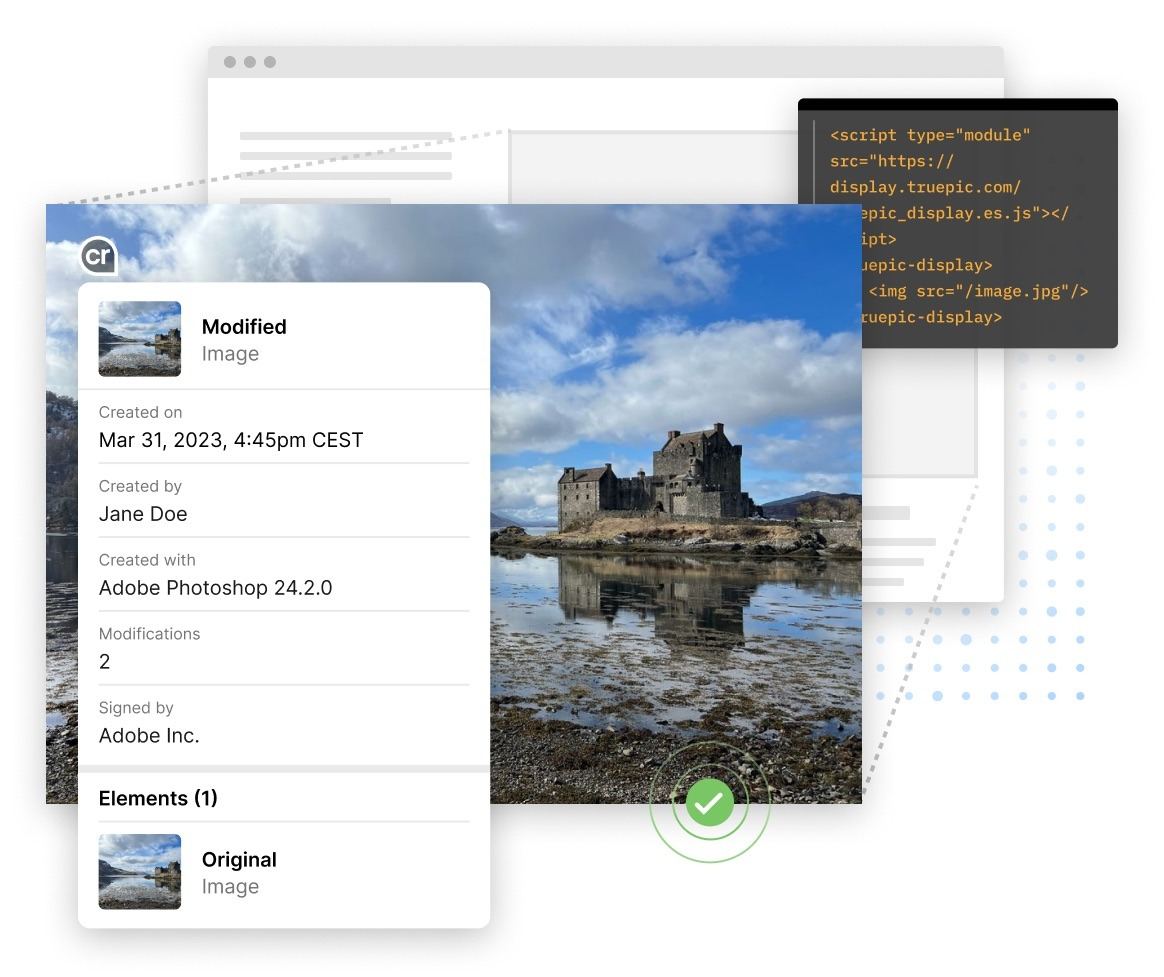
This was clearly created in Adobe Photoshop
The verification process is based on public-key cryptography similar to the protection used in web server certificates for establishing a secure HTTPS connection. The idea is that every image creator — be it Joe Bloggs with a particular type of camera, or Angela Smith with a Photoshop license — will need to obtain an X.509 certificate from a trusted certificate authority. This certificate can be hardwired directly into the camera at the factory, while for software products it can be issued upon activation. When processing images with provenance tracking, each new version of the file will contain a large amount of extra information: the date, time, and location of the edits, thumbnails of the original and edited versions, and so on. All this will be digitally signed by the author or editor of the image. This way, a verified image file will have a chain of all its previous versions, each signed by the person who edited it.
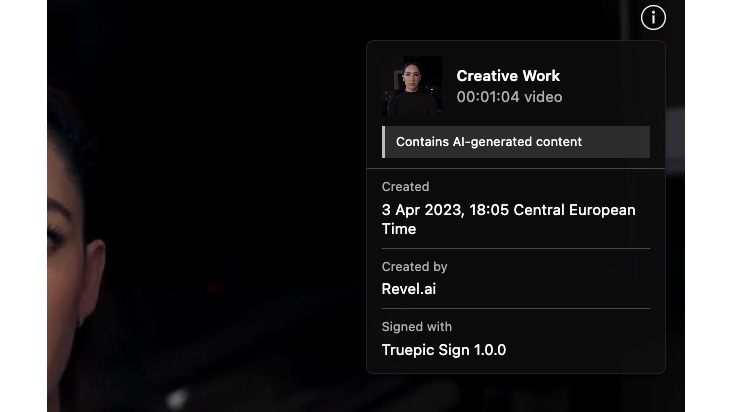
This video contains AI-generated content
The authors of the specification were also concerned with privacy features. Sometimes, journalists can’t reveal their sources. For situations like that, there’s a special type of edit called “redaction”. This allows someone to replace some of the information about the image creator with zeros and then sign that change with their own certificate.
To showcase the capabilities of C2PA, a collection of test images and videos was created. You can check out the Content Credentials website to see the credentials, creation history, and editing history of these images.
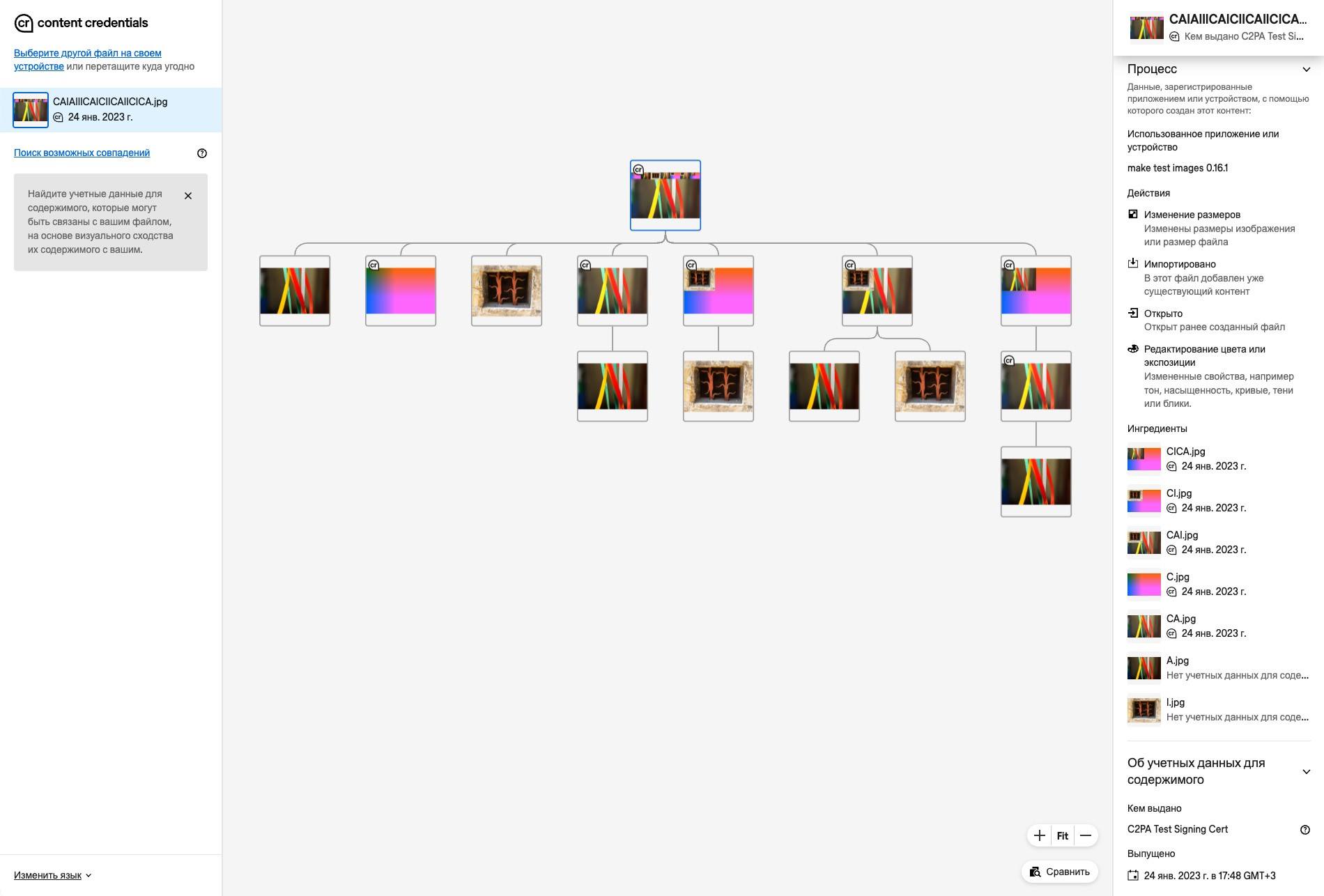
The Content Credentials website reveals the full background of C2PA images
Natural limitations
Unfortunately, digital signatures for images won’t solve the fakes problem overnight. After all, there are already billions of images online that haven’t been signed by anyone and aren’t going anywhere. However, as more and more reputable information sources switch to publishing only signed images, any photo without a digital signature will start to be viewed with suspicion. Real photos and videos with timestamps and location data will be almost impossible to pass off as something else, and AI-generated content will be easier to spot.